Forward Thinking About AI 'Big Error' and Your Employees
Companies can be proactive in their communication and understand what serious problems lie ahead when AI falls short of expectations
Errors aren’t always costly when understanding, trust or uncertainty are present yet that doesn’t mean that they can’t suddenly, shockingly become highly problematic when surprising, disappointing or upsetting ones materialize.
Many people are excited about AI’s presence, development and implementation, while others are more skeptical, cynical and resistant. Trust matters to that second group. AI has to prove itself to them, over and over, consistently, to earn and maintain credibility.
They may be scrutinizing the quality, ready for mistakes, to satisfy confirmation bias about the technology and it’s ever-increasing growth in use.
Recent research from the University at Buffalo School of Management “reveals that framing the competence of AI systems can significantly influence user perception, yet that only goes so far if those systems fall short of promises and expectations,” wrote Kevin Manne, the associate director of communications at the college.
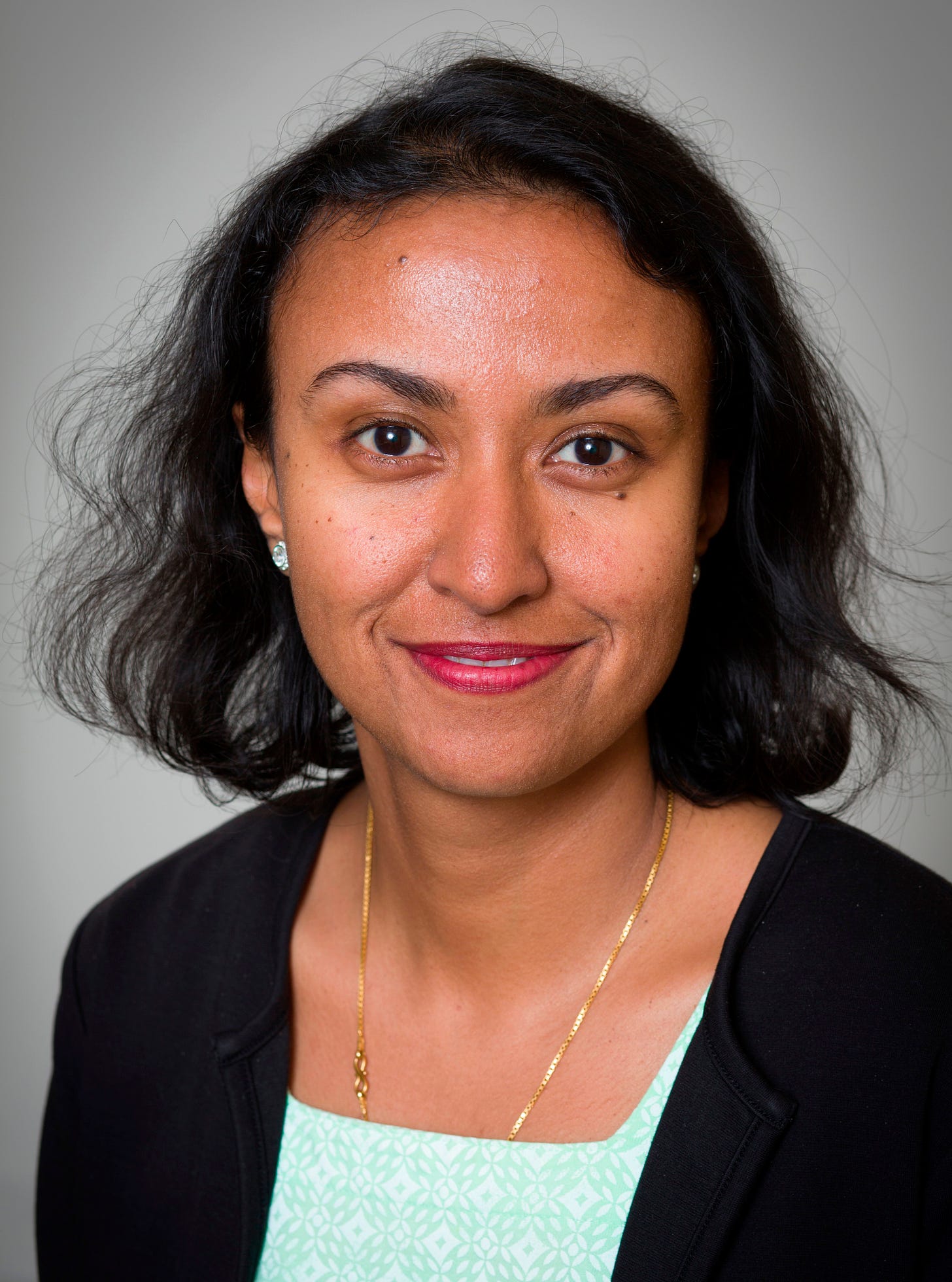
It’s a point that Sanjukta Das Smith, PhD, the chair and associate professor of Management Science and Systems at the UBSM, talked about with Manne.
“If AI makes a small mistake, users tend to be more forgiving — especially when it has been framed as competent,” Smith said. “But when AI makes a major mistake, trust plummets, and no amount of positive framing can recover it.”
That particular finding maybe was to be expected.
“This is not terribly surprising to me,” Smith tells Communication Intelligence, “as the condition that we tested for in this specific case was that of a major error. We are now digging into this phenomenon further at the moment to see if people tend to blame AI more than the analyst using it, or vice versa.”
The study showed how emotions and psychology are factors determining confidence and acceptance.
“A portion of the public might have a fragile notion of trust in AI to begin with, and so a major mistake ends up doing irreparable damage,” Smith says. “We also suspect algorithm aversion plays a role here: some people instinctively distrust algorithms, regardless of prior performance.”
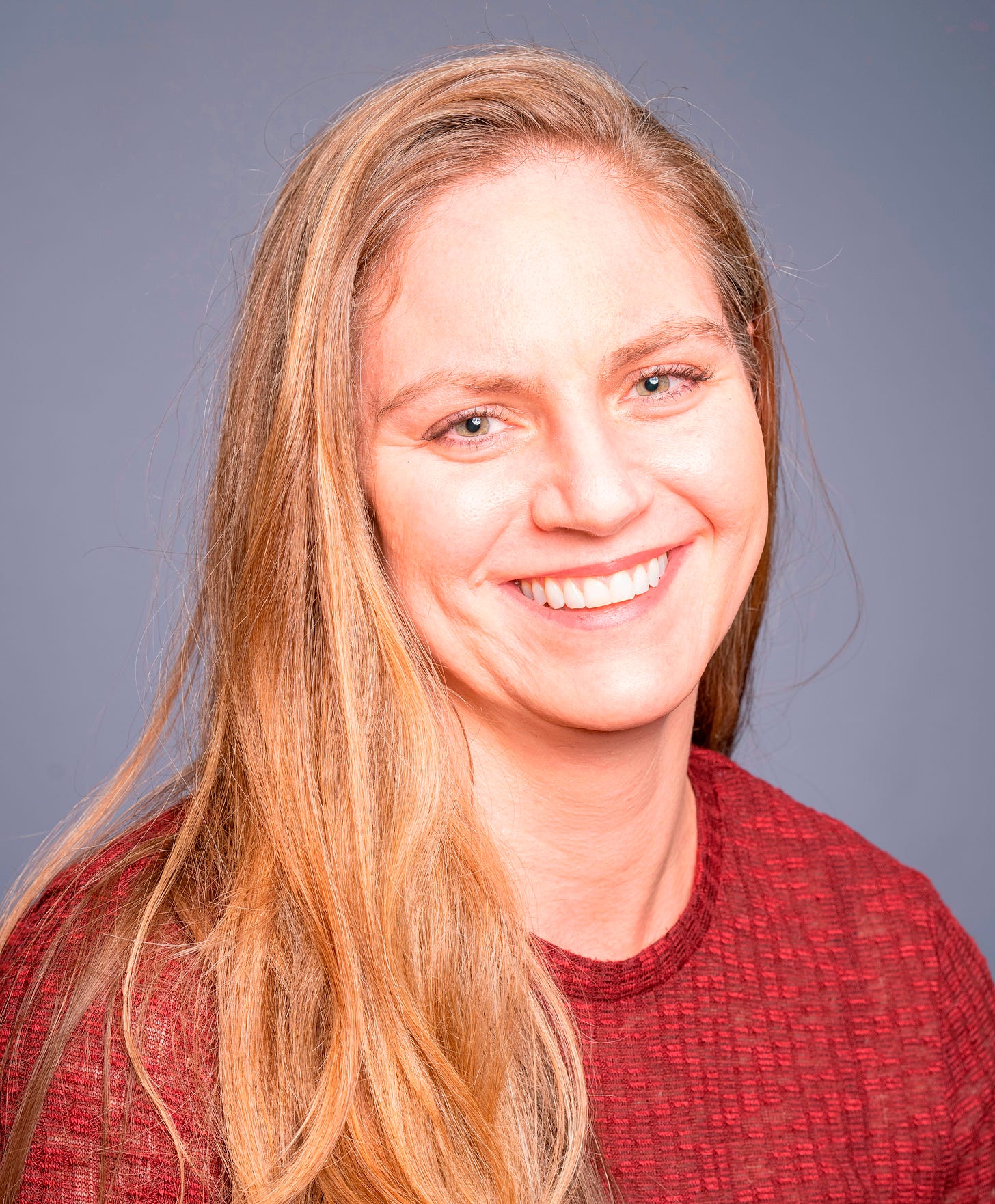
Organizational leaders should know that the technology won’t likely gain simple, full acceptance from everyone, at least at first.
“Employees won’t automatically trust AI, even if it’s widely used,” stated Laura Amo, PhD, assistant professor of Management Science and Systems at the school and a collaborative author of the research findings, “but emphasizing the reliability and accuracy of AI can encourage the adoption of the technology.”
“Effective communication about AI capabilities should be coupled with genuine competence to ensure long-term trust and user error tolerance.”
With its powerful strengths, potential and the excitement surrounding its use, it’s recommended and wise for leaders to pause and consider how they will protect their — and the AI’s system they utilize — credibility, by not exaggerating and overpromising its technological competencies.
“It’s important to not overhype the capabilities of what AI can do,” Victoria Gonzalez, a PhD candidate at the school and fellow researcher and author on the study, told Manne, ”because if employees feel misled about its capabilities, trust will be difficult to rebuild.”
Being forthright in persuasion regarding change management will benefit buy-in and the employer-employee relationship.
“Training employees on how AI works and why occasional mistakes happen can help prevent trust from collapsing after errors,” Gonzalez told Manne.
Precisely what constitutes a major mistake becomes an important question.
“Since we are looking at a purely professional — office work — setting,” Smith says, “an error would constitute scenarios where the AI’s output shows significant conflict with, or runs in opposition to, the ground truths, such as market conditions, etc., or new information that has come to light.”
AI, like human thinking, cannot be relied on as being above doubt and question.
“The thinking here is that no model is perfect, and often we discover these errors, minor or major, only after the model has been deployed in the wild,” Smith explains. “While minor errors are inevitable in any model, major discrepancies raise red flags that can cause trust to collapse.”
She speaks to a vital focus of leaders when depending on AI in their organizations.
“The lesson from our study has more to do with managing expectations of the users or consumers of these model results,” Smith details.
“Companies may need to focus on supporting employees’ AI literacy and cultivating employees’ broader analytical thinking as means to develop more pragmatic users of these models.”
She elaborates as to the value of this time investment and approach.
“If employees understand how models work and why errors happen, they’re less likely to overreact when mistakes occur,” Smith explains.
Once a major error happens however, gaining patience, some benefit of the doubt and time to mitigate distrust and uncertainty while making improvements, could be challenging.
“The experiments we have run so far show that the positive effects of ‘promoting’ AI to the users affect their initial trust in AI and their intention to act in accordance with what it suggests,” Smith says. “That effect, however, disappears once errors come to light.”
Thinking more about it, her professional gut instinct tells her something.
“My suspicion is that post-hoc explanation of major errors may run the risk of coming across as excuse-making, especially to those already wary of algorithms,” Smith says.
Fearful, impulsive leadership reaction or short-sighted, measured response leading to communication that gives off that impression is unlikely then to be helpful.
“A better approach,” Smith contends, “is to be proactive in training all employees in at least foundational AI literacy, so that knee-jerk reactions to errors are avoided when they do happen.”
She elaborates on how this can be a better plan and priming of the mind.
“These types of training may allow them to hold more measured, pragmatic viewpoints, considering the performance of the model in the past,” Smith forecasts.
“Still, some individuals may draw a hard line after a major error and view any explanation with skepticism, making it all the more crucial to build resilient trust from the outset.”